How Data Analysts Can Improve Business Decision Making
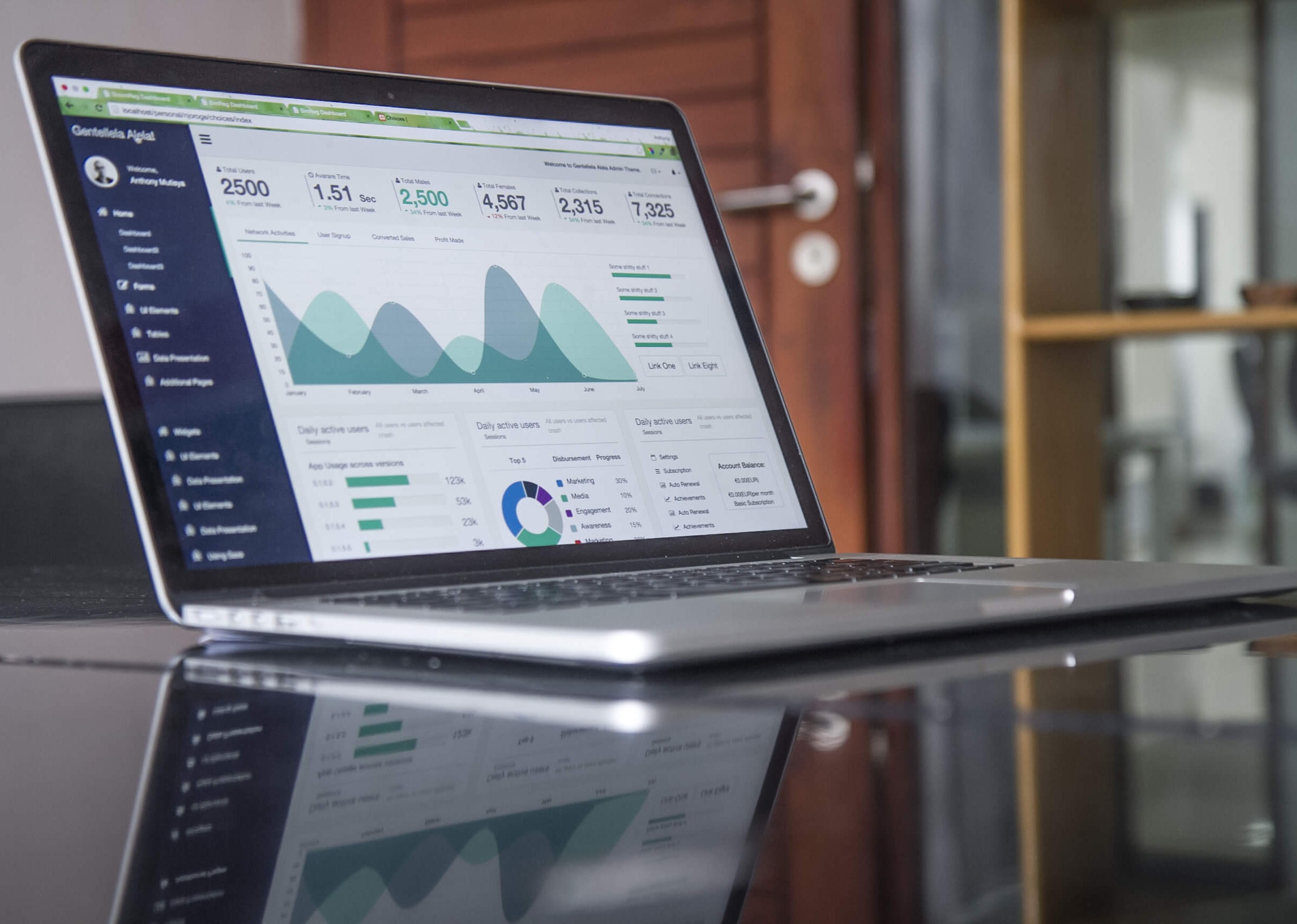
This blog post concludes a trilogy of articles about different specialists in the Data Science field we work with at expertlead by focussing on the role of Data Analysts. It will bring to light their distinguishing characteristics in comparison to Data Scientists and Data Engineers as well as demonstrate which core skills and respective tech stacks can be used to successfully perform their role.
Data-Driven Decision Making
While Data Engineers are involved in preparing and maintaining complete data architectures; Data Scientists in its complex analysis, organization and interpretation - Data Analysts, in turn, are mostly focused on finding patterns in numeric data and use it to help companies make better decisions. Data Analysts do not only bring value to the business by finding answers for the precise questions, but also by communicating the findings that provide a solid background for many business decisions, covering all aspects of work, e.g. monitoring and analysis of performance indicators, product or process metrics’ analysis, forecasting of growth potential etc.
Generally speaking, data search & cleaning, data analysis and data visualization represent three main fields of performed tasks. Same as for Data Engineers and Data Scientists, there are many variations of job titles in the Data Analytics area, including Business Intelligence (BI) Analyst, BI Consultant, Operations Analyst, Database Analyst, Business Analyst, etc. Despite the fact that there may be differences between particular types of specialists, what they have in common is the data-driven approach to the decision making process, including progress tracking and optimization on various levels: company, department, and personnel.
The Data Science Team Setup
We hear the buzzword Business Intelligence (BI) used often and the previously mentioned data driven approach is closely related to this. But what do we understand by BI? As other authors mention, it is an umbrella topic which in fact incorporates many frameworks and methodologies focused on getting fact-based solutions to enhance decision making within a company. This ranges from Key Performance Indicator (KPI) reporting to advanced statistical models. As previously discussed, specialists in the overall Data Science field usually support each other when working in a team, which means different stages of the data life-cycle should ideally be covered by different roles. Therefore, the Data Analyst can be supported by the Data Engineer in data acquisition and preparation. Meanwhile, Data Analysts themselves can assist Data Scientists in data analysis, visualization and presentation. Though ideally there should be a chain of interactions and dependencies, as there is always room for exceptions: perhaps there is no other type of data specialist in the team and thus everything should be performed by a single person, or data manipulation boils down to just dashboard reporting for a C-suite and there is no need for a Data Science team.
Since the scope of tasks varies on the company’s data, as well as the experience level of the specialists, questions that business stakeholders may be interested to find answers for can be:
- How can data analytics help in launching new campaigns / entering new markets and how can one keep track of it?
- How can employees’ productivity be optimized?
- How can one better understand which customers (demographics) to address?
- What are the main triggers of a company's growth / downfall or what trend will the company have within the next couple of years?
Therefore, in order to find an answer for the posed question, Data Analysts can turn to one or several of the following areas and tasks:
- Data acquisition & Maintenance: e.g. conduct research of potential relevant data sources (both internal and external) that can be used to identify trends and find a suitable strategy to collect acquired data
- Data cleaning: e.g. cleaning and organizing raw (unstructured) data
- Pattern identification & Analysis: e.g. using descriptive statistics to get a big-picture view of the data
- Optimization of statistical efficiency & Quality: e.g. improve and execute new analyses for different stakeholders
- Statistical analysis & Data interpretation: e.g. analyze interesting trends found in the data
- Reporting & Data visualization: e.g. create accessible and meaningful data visualizations, dashboards and reports
- Communication: e.g. present the results of the analysis to business stakeholders, including internal teams
Depending on the company's specific needs, more precise practical examples may include:
- Conceptual design and implementation of dashboards for different teams, e.g. to manage marketing campaigns
- Implementation of basic web tracking in connection with analytics tools
- Data modelling and creation of reports to get insights about the relationship between e.g. customer satisfaction and customer journey
- Development of a KPI framework e.g to measure product performance
- Analysis of the Up- and cross-selling activities, market basket, Social Media / CRM tools, customer churn & prevention and many more.
Professional Experience And Educational Background
Therefore, due to the breadth of the prospective requests coming from business peers, there is no set must-have tech stack for a person to be called a Data Analyst. This is also due to the fact that past professional experience, meaning the tool set used in a previous company, and educational background can shift specialists’ focus towards one or more particular tools. However, below we would like to enumerate what our community Data Analysts have expertise working with:
MS Excel; Power BI ; Tableau; Looker; QlikView / QlikSense; Statistica; Google Analytics; Data Studio; Firebase; SQL; Cassandra; SAS; SPSS; R / Python or another programming language; ETL design tools; A/B testing; Web analysis software; CRM systems; HDFS; MapReduce; Spark; Hive |
Same as with Data Scientists, educational background can guide Data Analysts in one or another direction in this position. For instance, a degree in a quantitative subject, e.g. physics, mathematics, statistics or another field with a strong emphasis on quantitative analysis can be essential for a particular company, project or task. Nevertheless, the truth is that Data Analysts may come from various backgrounds, including Economics, Business Administration, Strategic Management, Business Informatics, or even Sociology and Political Science, etc.
Data Life-Cycle
Of course, speaking from a data life-cycle perspective we discussed before, it highly depends on the company, starting from their core business focus down to its Data Science team (if there is one) and particularly whether there is a Data Engineer in place. The reason behind why the latter is important, is the fact that Data Analysts may or may not be deeply involved in getting well-organized and relevant raw data. It is important to understand that from the data perspective and from the beginning of the analysis, techniques used and expertise brought by Data Analysts can be very different. In case there is a huge amount of unstructured data coming from both internal and external sources which should be cleaned and organized for further analysis, it is important to keep in mind that a Data Engineer position will suit better for this task. On the contrary, if a startup is willing to get valuable business insights by following a data-driven approach, an experienced Data Analyst will not only be able to retrieve relevant data, create insightful reports and dashboards. He or she can also help set up a suitable process of data collection and the integration of different data sources as well as be in charge of maintaining data systems and databases.
Despite the fact that both Data Science and Data Analytics (incl. BI) fields are aimed to support business decisions through data, they are not the same. Therefore, it is important to stress the difference between Data Analyst and other fields of specialists. Data Analysts, same as Data Scientists, can be involved in performing predictive analytics and producing reliable foresights. However, the major focus of Data Analysts is still on understanding present and past prospects by finding patterns and trends in data in order to translate outcomes into business context. Thus, building complex models to make predictions is usually not their area of responsibility, same as Data Scientists’ high-level expertise in data interpretation (e.g. by training and optimizing Machine Learning models) or Data Engineers’ programming and software development abilities.
Many experts in the field agree that the distinction of the two roles lies in the type of question they aim to tackle: whether it is a precise business request (Data Analyst / “known unknowns”) which implies working mostly with static and structured data, or open questions without any known formula behind it, entailing the use of high-speed, complex and multi-structured data (Data Scientist / “unknown unknowns”). It is also believed that Data Analysts usually explore data from a single source like CRM systems, while Data Scientists examine data from multiple disconnected origins. However we believe it is not necessarily true in many cases.
Conclusion:
Therefore, any organization that utilises data needs Data Analysts. However, since companies vary greatly in many factors, Data Analytics methods, tools and strategy in general are quite diverse. It is crucial to keep in mind that Data Engineers, Data Scientists and Data Analysts are not in the same categories - they have many common aspects, but expectations, methodologies and approaches to their work as well as other people involved may be very different. Moreover, a very experienced Data Analyst may have a much wider scope of tools and techniques (s)he can apply in comparison to a junior specialist. Most importantly, in addition to a set of tools, technologies and software that they may need to master, among the top skills Data Analyst should possess are good understanding of business processes, communication skills and storytelling which are often de-emphasized. Moreover, it is crucial to understand which domain of knowledge a Data Analyst should have in order to get relevant leads since there are many types of data (e.g. marketing or operations) as well as its analyses (e.g. descriptive or predictive) that vary from industry to industry.
At expertlead we have a Data Analyst community of vetted freelancers. If you are interested in finding the perfect Data Analyst for your project, be it a specialist with a strong business domain knowledge and statistical background or someone who is able to provide an end-to-end BI solution, feel free to reach out to us.
Sources:
Jun 2020 - 7 min read
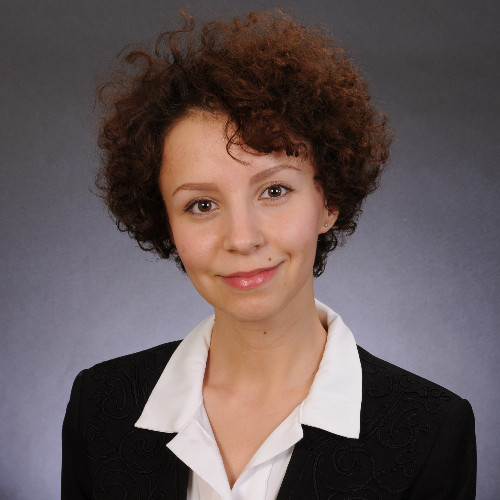
Anastasiia Prokhorova
Senior Freelance Manager