Data Engineers and What They Do
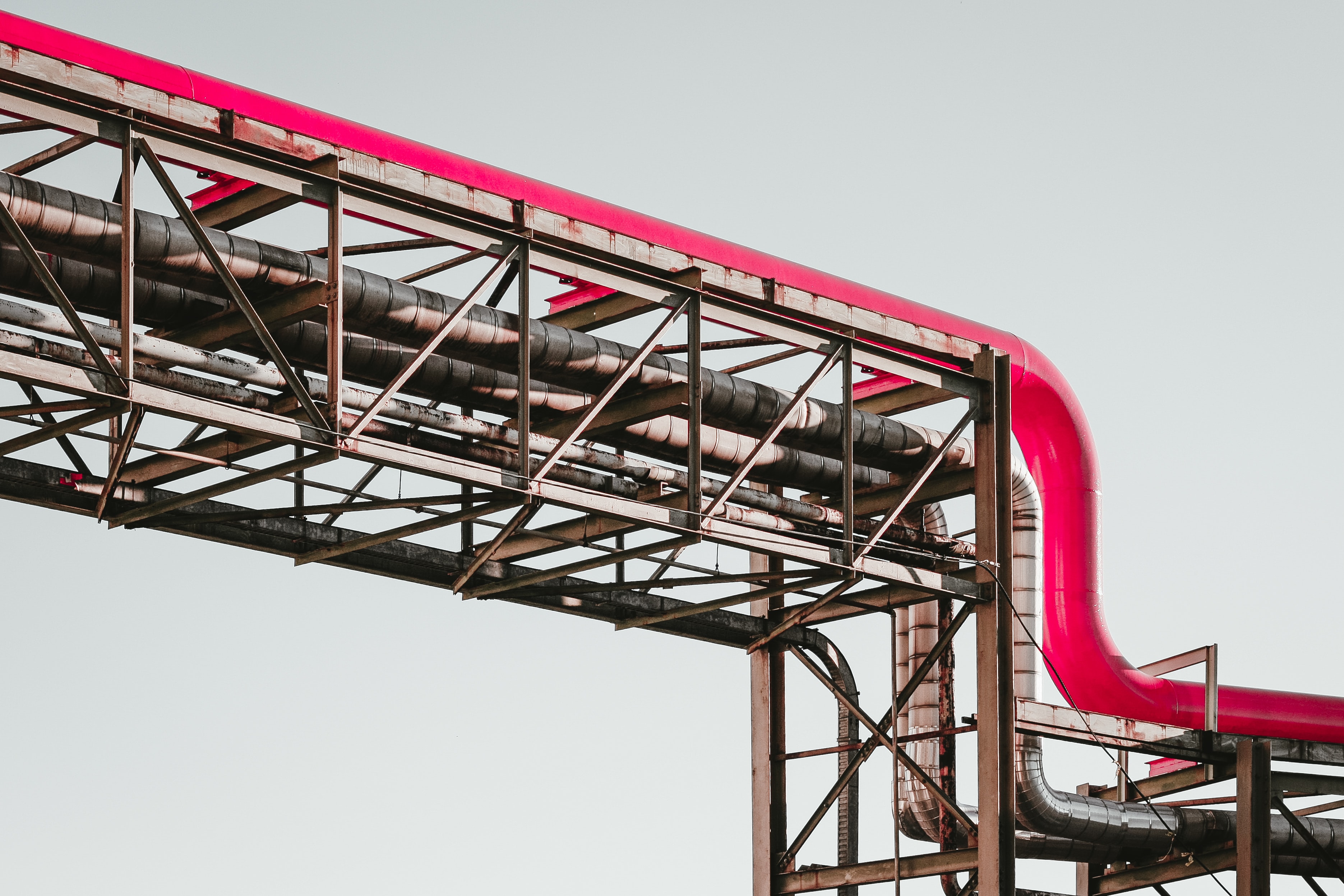
Your company has grown and with it the amount of data you produce. Your data infrastructure is struggling to provide you with clean data that allows you insights into your company's performance. You need a Data Scientist. At least that is what you think. In reality the Data Science field is very complex and split up into numerous different specialist roles each with their own capabilities and focus.
If you are looking for someone to help you set up a reliable infrastructure capable of collecting and optimizing relevant data, then you are looking for a Data Engineer. This article will let you understand the role and skills of a Data Engineer. It provides ideas about who these specialists are, what tasks they perform during their daily work and which tech stack they use in order to help other stakeholders, such as Data Scientists, Data Analysts and Business peers in general to get valuable insights from data that lead to a project's success.
Machine Learning and Artificial Intelligence are among the top fields that companies are interested in adopting into their current activities in order to get better predictions and understanding of the market. This results in increased value as well as income coming from more intelligent products and services. Nevertheless, since data science is a very complex field, many people are lost in identifying the exact place of these two popular notions in the data hierarchy. In fact, ML and AI are actually on the top of all processes that enable them to happen.
Therefore, before we are ready to implement deep learning techniques, we have to work extensively on data acquisition and data preparation, including the set up of its infrastructure.
In relatively small companies, there’s quite often only a single person who is dealing with everything: data engineering, data science and data analysis. However once an organization becomes bigger, as well as the scale of its projects, there is a need to distinguish the roles from each other.
Since one person cannot cover all the topics of the data science field, there exists a special category of dedicated specialists known broadly as Data Engineers, who are able to work with both structured data such as dates, addresses and names; As well as unstructured data, for instance, text / audio / video files or images in order to help businesses to get the most out of the foundational layer of the data life-cycle. We say “broadly” due to the vast amount of specialist titles in the Data Engineering field, for example: Data Architect, Database Administrator or Engineer, Big Data Architect/Engineer, BI Engineer.
In order to avoid confusion before we go deeper into the specificities of their job, let us first understand what background they have. From our experience looking through the expertlead community pool of data engineers, we can say that they have mostly been studying Computer Science, Computer Engineering or Software Engineering. Therefore, we can see that in comparison to Data Scientists, there is no need to have a deep understanding of scientific or academic fields such as statistics or mathematics for instance. Since the data science field and data engineering are not old concepts in the way they are presented today, we can see that specialists who have been involved in backend development or engineering, and/or worked on large scale architectures, can transit relatively easily into a Data Engineer position.
Now let us deep dive into specifics of their tasks and responsibilities. Since data is everywhere and can be of different types, the first step will be to set up the process of data gathering and understand how this data can be cleaned and stored, before it can be analyzed and put into production.
This process usually begins with a data schema, a so-called list of all the collected data which then leads to the next step of identification where this raw data should be stored - to identify a reliable data warehouse. The ability to design, develop and maintain a data warehouse is in high demand nowadays since it may support other activities, be it BI or Online Marketing. Another important aspect to keep in mind for Data Engineers is whether a data warehouse has to be an in-house / on premise server, cloud-base solution or hybrid data storage infrastructure, which depends on the data type and its amount, as well as the company's financial capabilities. Then comes the design of the ETL (Extract, Transform and Load) or ELT processes (swapped approach e.g. for data lakes) to ensure that data is getting into the data warehouse smoothly. Therefore, these three fundamental steps represent how Data Engineers design and implement data pipelines in most cases in order to ensure that raw, unstructured data can become analysis ready.
Donal Tobin, CEO at Xplenty, provides a good analogy to better define the role of Data Engineers:
If data scientists are train conductors, data engineers are the architects/builders of the railways that get the trains from A to B. Let's say the train conductor wants to deliver a payload somewhere that doesn't have an established railway. The conductor needs the railway architects/builders to connect the train to the new destination. The railway architects will study the terrain. They'll decide if it's better to go around, over or tunnel through mountains. They'll build bridges over rivers. They'll use all the tools available to build a railway that connects the train to the new destination. (Link to source)
Speaking about data engineering and the variety of roles in the field, we can’t ignore the Big Data concept mentioned above since this is what big technology companies such as Amazon or Netflix are flooded with. Characterised by high volume, velocity, and variety, Big Data and its engineering or architecture is not the same as “traditional” data handling with data warehousing. It is a data lake that is capable of handling this type of data and the ELT process mentioned before.
Therefore, since Data Engineering includes many steps and different kinds of activities, this process may require various data manipulation and management tools suitable for the task. Core skills and respective tech are as follows:
*Please note that this table is neither exhaustive nor a required list of skills and tech required for this position in each single case.
In conclusion, to become a Data Engineer is not easy, particularly when taking into consideration many of these specialists get their most relevant experience in real world practice rather than in academic research or online courses. Though if you are coming from a Computer Science background or Software Engineering, it is easier. With the growth of data and popularity of AI, Data Engineers will be in huge demand in the industry and will be a rewarding career opportunity for anyone willing to take it. And for us stakeholders, it is important to keep in mind that data engineers are very important for projects in the data science area since they prepare, build and operate the organization’s data infrastructure, setting it up for the following analysis done by data analysts and scientists.
At expertlead we have a Data Science community of vetted freelancers. If you are interested in finding the perfect Data Engineer for your project, feel free to reach out to us via this form.
Sources used:
Want to Become a Data Engineer? Here’s a Comprehensive List of Resources to get Started
What is Data Engineering: Explaining the Data Pipeline, Data Warehouse, and Data Engineer Role
Job Comparison – Data Scientist vs Data Engineer vs Statistician
Data Engineering: What Does a Data Engineer Do? How Do I Become One?
Apr 2020 - 6 min read
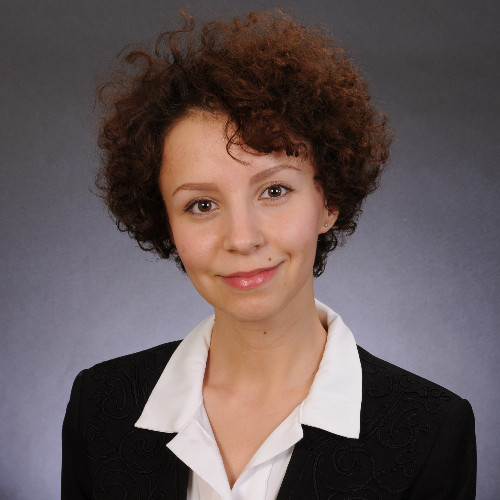
Anastasiia Prokhorova
Senior Freelance Manager